The adoption of AI could reinforce profit-centric thinking – or unlock a more cooperative and sustainable approach to tackling complex business and social issues.
The rise of GenAI models introduces additional complexities for responsible and sustainable decision-making. These systems are often trained on historical data that reflects profit-only criteria for decisions. As a result, there is a risk that the adoption of AI will perpetuate outdated paradigms, hindering the efforts to shape a better economy, society and environment. Unchecked, AI could reinforce historical biases – but if leaders integrate the principles of cooperative advantage, the adoption of GenAI could yet help re-orient decision-making toward a purpose of cooperative advantage for all stakeholders.
The concept of cooperative advantage
The traditional management literature, part of which was injected during the pre-training of foundational language models like OpenAI’s ChatGPT, is rooted in a corpus of knowledge from classic 20th-century scholars. These scholars, primarily grounded in economics and classical sociology, often depicted humans as individualistic and transaction oriented. But business paradigms are evolving towards stakeholder capitalism and the concept of cooperative advantage, not least because of recent shifts resulting from the pandemic and various other socio-environmental crises. (See ‘Cooperative Advantage: Rethinking the Company’s Purpose’, Prieto and Phipps, MIT Sloan Management Review, 2020.)
This emerging approach prioritizes individual and community well-being, drawing on the ubuntu philosophy – ‘I am because we are’ – a tenet deeply embedded in African American management history and cooperative traditions (African American Management History, Prieto and Phipps, 2019.) The approach advocates that businesses should aim for more than mere profitability; they should also consider the well-being of all stakeholders and members of their extended professional network. Based on principles of care, community, meaningful dialogue and consensus-building, cooperative advantage aligns business success with social good, benefiting employees, customers and the wider community, which in turn supports the long-term health of the business.
A notable example is Charles Clinton Spaulding. He led the North Carolina Mutual Life Insurance Company in Durham, North Carolina’s Black Wall Street during the Golden Age of Black Business, in the first half of the 20th-century. Drawing from these lessons, modern businesses can blend commercial success with local community benefits. One virtuous example is US fashion brand Kate Spade’s partnership with a for-profit, worker-owned supplier cooperative in Masoro, Rwanda, that now employs over 250 women and helps drive community development.
However, despite the exceptions from the past, and the rising interest in cooperative business models, the vast majority of practices and examples featured in management literature are still under the influence of individualistic and utility-maximizing theories. This systematic oversight poses a challenge: how do we embed cooperative advantage into decision-making, an arena increasingly shaped not only by humans, but also by pre-trained language models?
Cooperative advantage in the age of AI
It is crucial to expand the concept of cooperative advantage into the realm of AI-assisted decision-making. To achieve this, we’ve crafted and tested a new framework. This framework draws inspiration from the new role of human judgment in the AI age (‘Good Judgment Is a Competitive Advantage in the Age of AI’, Farri, Cervini and Rosani, Harvard Business Review, September 2023), to establish responsible boundaries for human-machine interactions and ensure the promotion of sustainable decisions.
Leveraging the capabilities of GPT-4, we applied and simulated the framework on real scenarios involving complex issues and multiple stakeholders, such as water infrastructure accessibility in India, or the sourcing of rare materials for electric vehicles from mines in Africa. Selected excerpts are reported in this article. The approach has three pillars: Context, Dialogue and Decision.
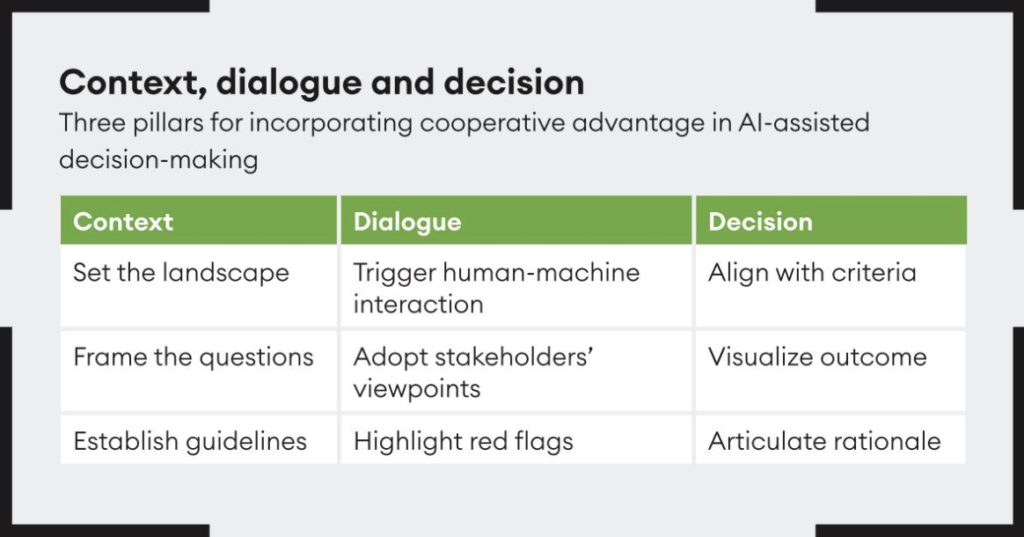
Context
Set the landscape
Organizations should calibrate AI systems to align with the unique context of their ecosystem. While the machine might already possess access to publicly available company data (such as the regulatory framework of the industry, company sustainability reports, news, etc.), other information might be proprietary, such as the firm’s specific sustainability initiatives, detailed actions and targets.
Frame the questions
Construct a sequence of prompts that ensure discussions are guided by well-formulated questions. These questions should encompass diverse perspectives and elicit consideration of trade-offs.
Take the case of an electric vehicle (EV) manufacturer evaluating whether to invest in cobalt mining in Africa for EV batteries. They might begin with the instruction, “Who are the stakeholders involved, and what are their specific needs?” Next, evaluate the benefits and concerns for each group: “What potential benefits and concerns exist for each stakeholder?” We then look to identify risks: “What are the key risks associated with cobalt mining, and are there any critical red flags in our current approach?” Finally, we consider mitigation and alignment with collaborative criteria: “What actions can mitigate the identified risks, and how do these actions align with our collaborative criteria and the diverse expectations of all stakeholders?” This chain of questions ensures a comprehensive and balanced exploration of the multifaceted issues at stake throughout the human-AI dialogue.
Establish guidelines
Organizations need to set clear guidelines, incorporating boundary conditions and safeguards. These can encompass ESG targets and policies, and distinct criteria related to hiring, procurement, travel, product lifecycle footprint, and diversity and inclusion standards. Such guidance ensures the machine recognizes the parameters for decision-making in the firm’s specific context, and when trade-offs are appropriate.
For example, when considering an investment in high-risk countries, the machine will help investigate potential risks such as corruption, community disruption, labor conflicts, non-compliance and competitive obstacles. It recommends proper engagement of third parties through due diligence, transparent communication, establishing strong partnerships with local entities, and adhering to international standards of conduct. This approach helps in identifying potential issues early on and ensures alignment with the organization’s ethical and sustainability commitments, while also fostering a stable and cooperative business environment.
Dialogue
Trigger human-machine interaction
The machine should serve as a collaborative partner, posing relevant questions, challenging assumptions, urging users to reevaluate biases, presenting diverse viewpoints and emphasizing nuanced trade-offs. This approach refines users’ capacity for making sustainability-centric decisions.
Adopt stakeholder viewpoints
Set up the machine to emulate various stakeholder perspectives. By doing so, it can present a range of viewpoints, foresee potential objections and guarantee that decisions are in harmony with sustainability goals, replicating a comprehensive stakeholder engagement process. Additionally, models like GPT-4 can now browse the internet to gather real-time information and news about specific stakeholders.
Take the example of a water-scarcity project in India: the team identified stakeholders including the municipality, local households and water filter supplier. The machine recommended adding health experts and local community influencers, who had initially been overlooked, to broaden the initiative’s perspective. When AI is embedded in a workshop setting, it can boost brainstorming sessions with human participants.
Highlight red flags
The machine can pinpoint elements that might have been missed by humans, prompting consideration of additional potential issues and risks. This aids in early mitigation, testing of potential scenarios, and bringing attention to unnoticed biases or overly profit-focused perspectives. It ensures potential negative externalities are detected.
Decision
Align with criteria The machine validates that decisions conform to pre-defined criteria of cooperation. When faced with multiple alternatives, it orchestrates a prioritization process based on pertinent criteria and weights, always referencing the preset context and boundaries.
In the case of the water scarcity project, the problem was tested against a set of 10 predefined criteria on collaborative cooperation. It turned out that the proposal demonstrated strong fit on several criteria like care and value alignment. However, the machine highlighted opportunities to place greater emphasis on deepening meaningful dialogue across all stakeholders and structuring authentic local community inclusion in decisions, providing concrete examples of how this could be done.
Visualize outcome
Distill complex deliberations and discussions into more comprehensible outputs. For instance, one could create a table or chart that succinctly captures and illustrates the primary stakeholders, their concerns, and the associated trade-offs in one comprehensive visual representation. This aids in ensuring clarity and understanding for all involved.
Articulate rationale
The machine assists human users in framing a comprehensive and clear justification for the decision, ensuring its transparency, logical foundation, auditability, and shareability with a broader audience. It also integrates decisions with pertinent ESG metrics.
Using this three-step process, the collaborative journey between human and machine can ensure that the resulting decision is sustainable in its process, achieving outcomes that surpass current standards. This synergy demands both the user’s judgment and the machine’s structured guidance – a partnership that yields optimal results.
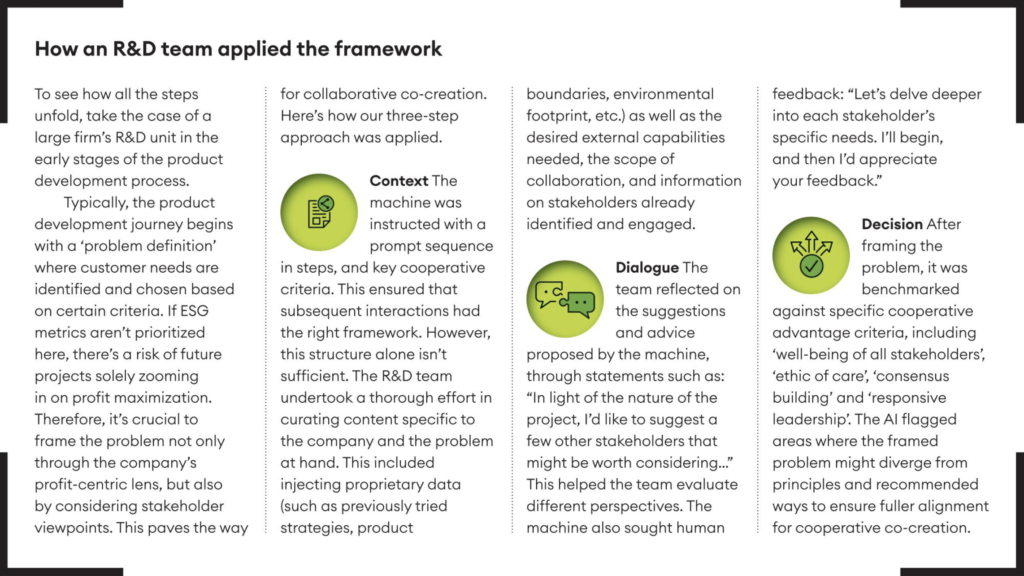
The path ahead
As the discourse around GenAI continues to evolve, it’s imperative to steer it towards a path that aligns with broader societal values and sustainable business practices. The framework discussed here provides a roadmap for achieving such alignment by fostering cooperative advantage: a paradigm where organizations transcend mere profitability to also prioritize the holistic well-being of all stakeholders, thereby creating a more equitable and sustainable business ecosystem.
Through the integration of cooperative principles within the AI-assisted decision-making process, businesses can not only enhance their operational efficacy but also contribute to a more inclusive and sustainable economic landscape. The articulation of cooperative advantage within the realm of generative AI is a promising step toward redefining how businesses can blend profitability with social impact, and usher in a new era of responsible and insightful corporate governance.
Leon Prieto is director of the Center for Social Innovation & Sustainable Entrepreneurship in the College of Business, Clayton State University. Simone TA Phipps is a professor of management in the School of Business, Middle Georgia State University. Paolo Cervini is vice president and co-lead, and Gabriele Rosani is director of content & research, at Capgemini Invent’s Management Lab.