How can leaders shape the journey towards becoming a data-driven organization?
Few companies question the value of data and artificial intelligence (AI) for their business. Many proclaim their aspiration to become a ‘data-driven organization’. But there is often some confusion about what precisely that means, and how best to achieve it.
Data-driven organizations are fundamentally defined by two key characteristics. First, a data-driven company considers data as a strategic asset, rather than an exhaust from its operations. Secondly, in a data-driven organization, most relevant decisions are informed by insights generated from data using AI.
And why is becoming data-driven so important? Simply because of the huge business opportunities presented by big data and AI. Opportunities include generating more revenues (doing more with the same), reducing costs (doing the same with less), or even creating completely new businesses. Moreover, all companies will do this; the ones who don’t are out.
Apart from the business opportunities, there are also huge social opportunities. Think about better monitoring and progress towards the UN Sustainable Development Goals, responses to natural disasters caused by climate change, alleviating forced migration, or the fight against Covid-19 (and future pandemics). And of course, big data and AI is not only for the private sector. It is equally applicable to governments and public administration. Governments around the globe have already included these technologies in their technological, educational, economic and sustainability programmes, and few citizens would disagree with the need for more evidence-based policymaking and less voting-based policymaking.
There are, however, some additional challenges for the public sector. Firstly, overall, public bodies lag behind the private sector in digital transformation – and without digital transformation, you cannot start a data journey. Secondly, not all public decision-makers embrace data by default as a source for taking decisions. From a data culture perspective, that’s critical: data is the cornerstone for transparency – but sometimes data might show things that are contrary to certain political agendas.
In any sector, from a leadership perspective, the process of becoming a data-driven organization is best viewed as a journey, rather than as a result. There is a progression from exploring the opportunity, to consolidating the data and transforming the organization, to realizing the benefits. The more an organization advances on the journey, the more data can be transformed into value in a scalable manner. In the later phases of the journey, full advantage can be taken of machine learning and AI.
As I explore in my latest book, A Data-Driven Company, there are four key phases to the data journey.
The data journey
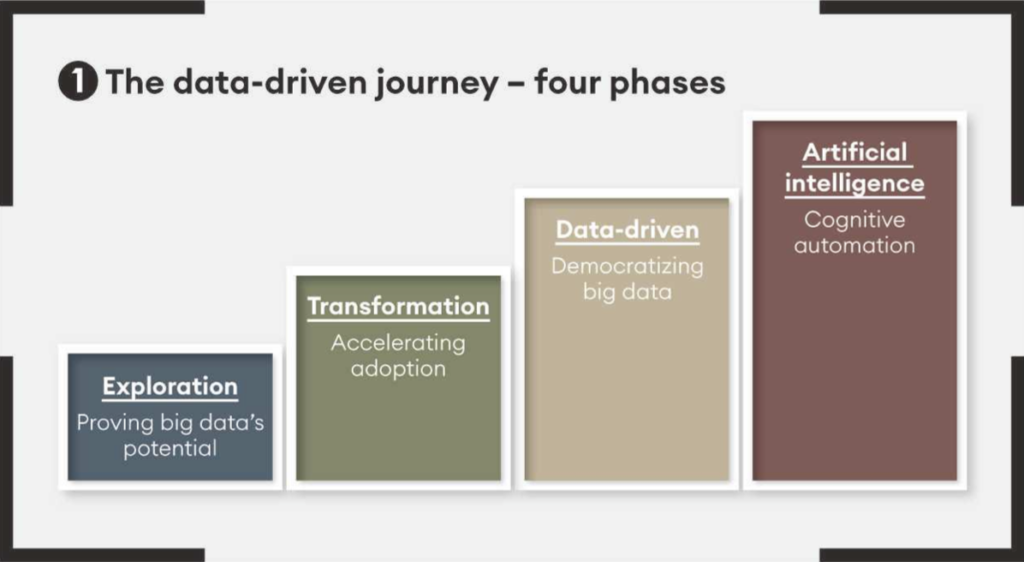
The first phase of the data and AI journey is one of exploration. This process can be started bottom-up, by data enthusiasts with technical capability – for instance, data scientists or data engineers – or top-down, by managers who’ve heard about the benefits of data for business. Either way, an existing business problem – also known as a ‘use case’ – is typically selected, such as reducing churn or increasing the effectiveness of marketing campaigns. Data is collected and analytics applied to see how data and AI could solve the problem.
The central objective of the next phase, transformation, is to prepare the organization to treat data as a strategic asset and create value from it in a systematic manner. Typical activities include the selection and implementation of strategic use cases which have significant impact on the business. It is also important to develop a data-sourcing strategy. Large organizations have a wealth of data, but collecting and storing it all in an accessible way is not trivial. Breaking down data silos is one of the less technical challenges that comes with the transformation process – leaders need to dedicate significant attention to this.
Being in the data-driven phase of the journey means that many of the important company decisions are now informed by data – that is, by data-rich insights, in addition to conventional wisdom, experience and intuition. It is in this phase that companies begin thinking about ways of deriving value from data in addition to internal value. For some sectors, insights generated from first-party data can create significant value for other sectors – such as mobility data from the telecommunications sector.
The final artificial intelligence phase is where full value can be created from the data, through analytics, machine learning and other AI technologies. Making maximum use of big data and AI also comes with new responsibilities, particularly related to privacy and the undesired consequences of AI and big data. Putting data and AI at the heart of an organization requires that leaders pay much more attention to keeping the data safe, not only for legal compliance reasons, but as a bedrock for establishing and maintaining customer trust.
Lessons for responsible use of data and AI
Moving forward on the data and AI journey requires huge numbers of decisions to be taken. However, many of those decisions end up being taken in an implicit and ad hoc manner. Leaders can accelerate the journey by taking those decisions in an explicit manner, evaluating the different alternatives along with their corresponding advantages and disadvantages.
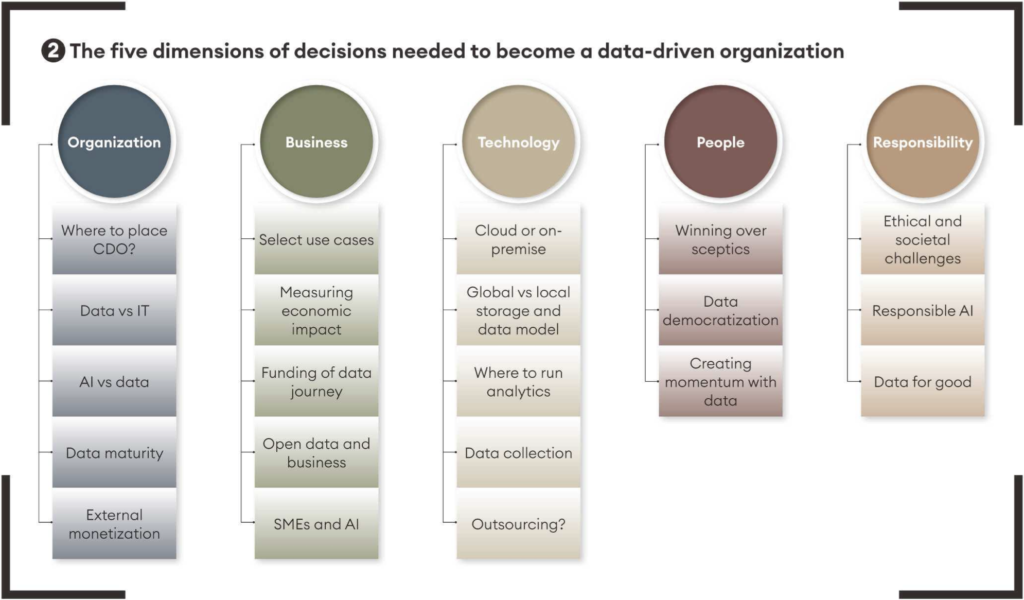
There are five dimensions of decisions that need to be considered (see graphic, above): these relate to the organization, business and finance, technology, people, and responsibility.
Organization is about decisions related to the organizational aspects of becoming data- and AI-driven. Questions that need decisions might include: where should we place the chief data officer (CDO) in the organization chart? How do data and IT live together? Is AI included in the data organization or separate? How will we measure data maturity? How will we go about external monetization of data?
Business and finance deals with the main business decisions related to data and AI, including: how will we select AI and big data use cases? How will we measure economic impact? How will we fund the data and AI journey? What is our approach to open data? What is our stance regarding small and medium-sized enterprises?
Technology provides lessons about the technological decisions that organizations will face during their data and AI journey. For instance: cloud or on-premise? Local or global data storage? Unified data model or not? Where to run the analytics, globally or locally? Do we need a data collection strategy? What are the pros and cons of working with external providers and partners?
People involves decisions about upskilling the workforce and having the right profiles on board to democratize the power of AI and data beyond a specialist data department. That doesn’t mean that each employee needs to become a data scientist. There are several tools that provide machine learning or natural language processing capabilities to non-technical people, which can solve maybe 60% of business problems. This relieves the dedicated, specialist data team from working on the business’s simpler problems, so they can focus on solving the hard and interesting problems – which helps keep team members interested and maintains their motivation (with a corresponding effect on retention).
The other lesson relating to people has to do with the common organizational resistance to change. Becoming data-driven requires that data be shared company-wide, while respecting confidentiality and all privacy and data protection regulations. Traditionally, data is stored departmentally; the department has control over the data and decides who has access to it. These are the notorious data silos. Data is power, and it is not easy to share power. Dealing adequately with sceptical people is therefore crucial for moving forward on the data journey.
One way to do that is to look for a champion in any ‘data-sceptical’ department, work with him or her on a data project under the radar, and then – once there are interesting results – let the champion present the results to the department. Change from inside is always easier to accept than change imposed from outside.
Responsibility reflects the increasingly widespread expectation that technology should not be used only to generate profit. More companies espouse care for their impact on the planet and on the societies they operate in. Yet despite their many benefits, AI and big data can have unintended, negative consequences. Who has not heard about facial recognition algorithms that discriminate against coloured people? AI systems that hire more men than women? Algorithmic decisions that are opaque, black boxes that no one understands? Autonomous decisions without proper human intervention or oversight? Or excessive automation substituting human labour?
Leaders need to deal with these ethical questions head on. Increasingly, organizations are adopting so-called AI principles or AI codes, which aim to ensure the good use of AI without the negative, unintended consequences.
The future of work and work of the future
Among all the concerns about AI automation, the fear that it will substitute human labour is perhaps the biggest. Many researchers have tried to estimate the impact of automation on employment: for example, Carl Benedikt Frey and Michael Osborne at Oxford University have estimated that 47% of American jobs could be at high risk of automation, based on detailed task descriptions for 702 occupations compiled by the US Bureau of Labor Statistics. Other researchers, however, argue that while tasks may be automated, whole jobs are unlikely to be – unless 100% of that job’s tasks can be automated, which is rare.
Whatever the case, automation by artificial intelligence will undoubtedly have a substantial effect on the future of work. Jobs will disappear, new jobs will appear, and the nature of existing jobs will change. Nobody can claim to know how these three phenomena will play out in the future. There is, however, consensus that jobs that require creativity, social interaction, and high skills are more difficult to automate than low-skilled and repetitive jobs.
Leaders have a critical role in steering the future of work, and the work of the future, in a positive direction. Unfortunately, many companies today wrongly assume that low-skilled jobs are filled with ‘commodity’ people, and therefore can be automated.
Analysis of US Bureau of Labor Statistics data by Gary Hamel and Michele Zanini reveals that 70% of US employees are in jobs deemed to require little or no originality (Humanocracy, 2020). Yet as they point out, “it’s a thinking error to assume that the vast majority of jobs in an economy offer little scope for the application of the uniquely human capabilities that distinguish people from machines”.
Hamel and Zanini conclude that what makes a job ‘skilled’ is not the nature of the work, but whether or not the workers have the opportunity to use their creativity, social skills and problem-solving capabilities. By empowering people to do what they are good at, visionary leaders can turn even apparent ‘dead-end jobs’ into creative, rewarding ‘get-ahead jobs’.
The future of business is data-driven. Leaders have a vital role in guiding their organizations on the data journey. But to truly succeed, they will need to simultaneously unleash human potential – and create a collaborative, rather than competing, relationship between human labour and AI.